This article was originally published by Mark Thompson at MakingFamilyHistory.com
Identifying the people in a photo or letter collection is a top priority for family archive projects. It’s incredibly frustrating to have a beautiful stack of old photos or precious family letters and not know who is in them.
Unfortunately, identifying people in old collections is easier said than done: last names may be omitted, nicknames could be used instead of formal names, and letters might lack dates.
In larger collections, this problem is reduced because people are mentioned many times by different people. Each time they are mentioned by someone new, a new clue may be found. Each mention provides a clue that may help identify them.
However, in large collections, comparing and analyzing these clues can be overwhelmingly complex. Could ChatGPT simplify this challenge?
Comparing Items
Comparing the information found in each item in a collection is a daunting task. Comparing items in a large collection is especially challenging and requires both art and science to succeed.
On the science side, a complete research log is key. I use a spreadsheet-based research log, tuned to the needs of each project, to capture facts and dates about each item. This makes it relatively easy to find relationships between items.
Here is a simple example to illustrate the spreadsheet I use:
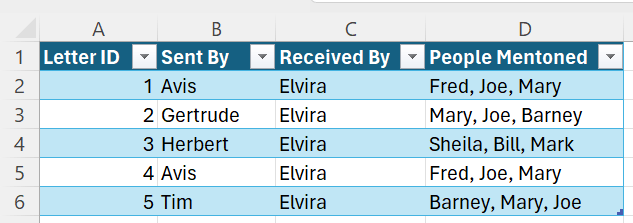
On the art side, a good memory for names, facts, and patterns is a huge bonus. Like many genealogists, I live for the “Eureka! I’ve seen that name before!” moment after a multi-hour research session.
As much as I enjoy the adrenaline rush of these eureka moments, I don’t like to rely on my memory to make them happen. Developing processes that make these patterns easier to find is crucial to successful research.
Clustering
Clustering is a technique for finding the relationships between groups of people or things. Even if they don’t use the term, genealogists use clusters every time they research. For example:
- The phone book clusters people who live in the same town into groups of people with similarly spelled last names.
- Land maps cluster people into groups who own land that is close together.
- The Leeds Method clusters people into groups who descend from the same grandparents.
- Census records cluster people into groups of people who lived in the same building at the same time.
- Friends, associates, and, neighbours (FAN) clusters group people together who are familiars of our family members.
Finding and understanding these clusters, and the relationships between the people in the cluster, can generate clues that are critical to breaking down genealogy brick walls!
The Manual Approach to Clustering
Before automating anything, it is important to learn how to do it the “manual” way.

To make it easier to identify clusters of people I start with a detailed research log. I track the names of each person mentioned in each item in the log. Then, using advanced spreadsheet techniques, identify the people who are mentioned together in the same items.
Knowing that these people are likely connected, I can then focus my efforts on determining how they are related. They might be friends, coworkers, or family. Knowing how they are related makes it easier to figure out who they are.
For large collections with hundreds of items and thousands of people, I use a network analysis tool named Gephi, to create diagrams for deeper analysis.
While invaluable, clustering with Excel and Gephi can be daunting initially. Even with a meticulous research log, this analysis technique can require 50 to 100 hours of practice to use with confidence.
How To Use ChatGPT To Simplify Clustering
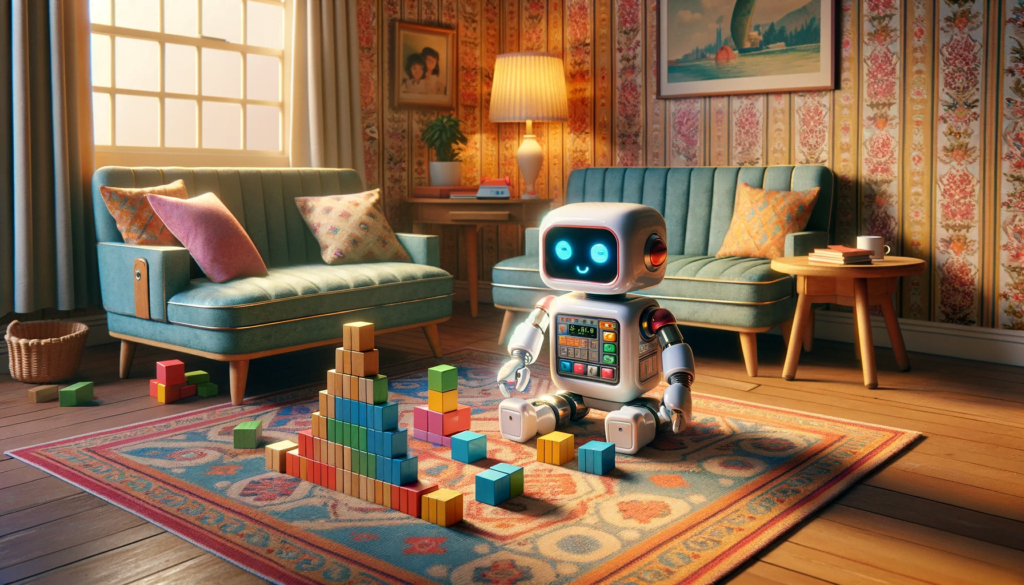
Getting Started: Prompt Planning In a Nutshell
When approaching a new problem with ChatGPT, consider the following steps:
- Determine how to provide the AI with the necessary information for the task.
- Decide the best role for the AI to assume when tackling the task.
- Define the specific task(s) you wish the AI to accomplish with the provided information.
- Choose the format in which you want the AI to deliver its findings.
Prompt Planning Example: Clustering People Mentioned in a Collection of Letters
I’ll walk you through the prompt planning process that I used with a real-world family archive project so that you can try this, or something similar, yourself. Note: The data mentioned in this article is sourced from an actual client project, with permission granted for its use here.
How to Provide the AI With the Information
As the archival information is stored in an Excel spreadsheet, the best option would be to find a way to use the existing spreadsheet directly in ChatGPT.
ChatGPT Plus added a feature today that was previously part of the “Advanced Data Analysis” plugin. This proved especially good timing for this example because ChatGPT Plus can now read an Excel spreadsheet directly. As such, there was no need to convert the spreadsheet to text, or a CSV file, to place it in the chat prompt.
Assign a Role to ChatGPT
Next, it is important to assign a role for the AI to adopt when performing the task. The role assignment is critical to do first as it sets the context for how additional instructions will be understood by ChatGPT.
As I was trying to perform a relatively complex data analysis task with archival documentation, I wanted ChatGPT to assume a role that specialized in this type of work. This would give it the context to understand the rest of the prompt.
Prompt: Please act in the role of an expert data analyst who has a deep knowledge of archive and records management.
Define the Task you Want To Do
After some testing, it became clear that there were several steps in this particular task that worked best when described separately.
Find the Information
First, ChatGPT needed clear guidance on how to find the information in the spreadsheet.
Prompt: Look for the column titled “People Mentioned” on the “Letters” tab in the provided Excel file.
Understand the Information
Next, it needed guidance on what kind of information it was going to work with. Ensuring that ChatGPT understood what it was working on, and not just where it was found, would make it possible to perform tasks on the information using natural language.
Prompt: This column contains a comma separated list of the people mentioned in a letter. Each row in the spreadsheet refers to a different letter.
Extract The Information
Once I had “taught” the AI how to find and understand the people mentioned in the spreadsheet it was time to get down to the real work. The goal was to identify the people who were mentioned most frequently in the letters and then to find the cluster of people who were also mentioned with them in the letters.
As this collection of letters is between family members, I hoped that the resulting clusters of people would know each other somehow and that the clusters would help identify them.
Prompt: Identify the five people who are mentioned most frequently across all the letters. Then, identify the five people who are most frequently mentioned in the same letter as each of the people you just identified.
Choose the format for the AI to Present Its Results
While there are a few common forms for a cluster analysis to take, the first approach I tried was in a table.
Prompt: The response should be a table, with the first column containing the most frequently occurring names, and the second column containing a comma-delimited list of the names which are most frequently found along with them.
The Final Prompt
After testing each step in the prompt plan, here is the final prompt.
Prompt: Please act in the role of an expert data analyst who has a deep knowledge of archive and records management.
Look for the “Letters” tab in the provided Excel file. Each row in this spreadsheet contains information about a letter. The column “People Mentioned” on this tab contains a comma separated list of the people mentioned in a letter. Each row in the spreadsheet refers to a different letter.
Identify the five people who are most frequently mentioned across all the letters.
Then, identify the five people who are most frequently mentioned in the same letter as each of the people you just identified.The response you provide should be a table, with the first column containing the most frequently occurring people, and the second column containing a comma-delimited list of the names which are most frequently found in the same letter with them.
The Final Prompt – Cluster Diagram Edition
Although a table format helps see who else is mentioned in the same letters as the most frequently mentioned people, there is a graphical format that provides additional insight called a Cluster Diagram.
Cluster diagrams show relationships not just between individual people, but between groups of related people. This additional layer can provide insights that are easily missed in a table.
This follow-up prompt was also modified slightly to present a larger cluster of people for analysis. However, a filter was used to limit the number of people in the chart so that it was easier to read. Simply said, I wanted to identify the people who had an ongoing relationship with the most frequently mentioned people.
Prompt: Please draw me a cluster chart that shows relationships between the people mentioned in the same letter as those you identified in the table. Draw a connecting line between two individuals if they are mentioned together in at least two letters. The line weight should be the same for all relationships.
Why This Cluster Diagram Is So Amazing!
It’s Useful For Research
The diagram clearly shows two clusters of people that are mentioned in the same letters as the most frequently mentioned people. And, the two clusters are connected by one individual, Elvira Caroline Ingersoll.
There is no need to go deeply into the family used in this example, but I will say that the cluster diagram is spot on! Elvira was a prolific writer and recipient of letters. In one generation, she was a daughter who communicated frequently with her siblings and parents. In the next generation, she was a mother who communicated extensively with her husband and children.
Any family historian or archivist would assume that this was a possibility if they saw this cluster chart. In this case, this clue would quickly put them on the useful track!
It’s Conceptually Easy to Create
It is difficult to understand the concept of a cluster and how to determine which information is beneficial to include within it. ChatGPT was able to understand my direction in natural language and retrieve the necessary information directly from a spreadsheet.
It’s Technically Easy to Create
Filtering a cluster down to the most useful items is technically difficult to do in Excel or Gephi. The fact that I got an easy-to-read and easy-to-understand chart so easily was nothing short of mind-blowing. This shows huge potential for “quick and dirty” cluster analysis, something that is relatively difficult for genealogists and archivists to do today.
Total Time Saved Using ChatGPT
The way I usually make these charts takes about 30 minutes of spreadsheet work and 60-90 minutes of work in Gephi. Keep in mind, this is after about 100 hours of learning and practice.
To create these charts in ChatGPT took only 30 minutes. Although there was some conceptual overlap with learning how to do this manually, there were none of the technical challenges associated with using complex, and occasionally buggy, pieces of software.
To be fair, Gephi can handle more information and more complex types of clustering and visualization than ChatGPT. While I will still need to use Excel and Gephi for more complex analysis, I won’t need Gephi for this type of quick and dirty cluster analysis anymore.
Navigating Challenges

Test Each Step Separately
This prompt involved several steps, and each step needed to be tested separately before it could be combined with the other steps. It was difficult to see where things were going wrong when I tried to test several steps at the same time.
New Chat Windows
I found it best to frequently start a new chat window during testing. Hallucinations and unexpected results were introduced when I tested several steps in the same chat window, particularly if something went wrong in a previous step.
The Importance of Clean Data
Starting with a clean, well-structured, research log is key to the success of any data analysis project. The old axiom of, “garbage in, garbage out” should never be forgotten.
Clustering with ChatGPT is about Insight, Not Tools
With ChatGPT, clustering becomes less about juggling complex software and more about spotting the helpful patterns in your data. Yet, there’s no silver bullet here; it still requires a knack for seeing the patterns in the first place.
Conclusion and Final Thoughts
When I started this article, I had high expectations for some parts and very low expectations for others. In the end, my expectations were exceeded in all areas!
Clustering is a very useful technique for identifying people in a family archive and determining the relationships between them. Although, it can be technically challenging to undertake this type of analysis.
ChatGPT had no difficulty accessing and understanding archival information in a complex Excel spreadsheet. Because of that, I had no difficulty interacting with the information in the spreadsheet using natural language.
ChatGPT made quick work of creating cluster tables and diagrams that showed the people most frequently mentioned in the archive. It also had no difficulty identifying the people who were mentioned in the same letters along with them.
Amazingly, ChatGPT could apply filtering rules to these clusters of people, a conceptually and technically difficult activity to do with traditional clustering tools. The ease with which ChatGPT produced clear, comprehensible cluster diagrams, a feat usually only possible through significant learning and practice, cannot be understated.
I am super excited about the potential of ChatGPT to analyze archives. Dozens of use cases immediately come to mind where it will greatly speed up my work.
My next ChatGPT clustering challenge though will come from the field of genetic genealogy … DNA Match clusters!
I’m very excited to hear what you think about using ChatGPT to perform cluster analysis. What do you want to learn from Clustering? What mysteries are waiting to be found in your family archive?
For Further Reading
- The Leeds Method for clustering DNA matches – Dana Leeds
- Creating Gephi Network Graphs of your DNA Matches – Family Locket
- Cluster Analysis – Wikipedia